This is an old revision of this page, as edited by 212.138.47.29 (talk) at 19:13, 13 March 2006. The present address (URL) is a permanent link to this revision, which may differ significantly from the current revision.
Revision as of 19:13, 13 March 2006 by 212.138.47.29 (talk)(diff) ← Previous revision | Latest revision (diff) | Newer revision → (diff)
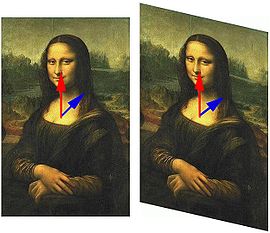
These concepts play a major role in several branches of both pure and applied mathematics — appearing prominently in linear algebra, functional analysis, and even a variety of nonlinear situations.
The German word eigen was first used in this context by Hilbert in 1904 (there was an earlier related usage by Helmholtz). "Eigen" can be translated as "own", "peculiar to", "characteristic" or "individual"—emphasizing how important eigenvalues are to defining the unique nature of a specific transformation. In English mathematical jargon, the closest translation would be "characteristic"; and some older references do use expressions like "characteristic value" and "characteristic vector", or even "Eigenwert", German for eigenvalue. In the past, the standard translation used to be "proper". Today the more distinctive term "eigenvalue" is standard.
Definitions
Transformations of space—such as translation (or shifting the origin), rotation, reflection, stretching, compression, or any combination of these; other transformations could also be listed—may be visualized by the effect they produce on vectors. Vectors can be visualised as arrows pointing from one point to another.
- Eigenvectors of transformations are vectors which are either left unaffected or simply multiplied by a scale factor after the transformation.
- An eigenvector's eigenvalue is the scale factor that it has been multiplied by.
- An eigenspace is a space consisting of all eigenvectors which have the same eigenvalue, along with the zero(null) vector which itself is not an eigenvector.
- The geometric multiplicity of an eigenvalue is the dimension of the associated eigenspace.
- The spectrum of a transformation is the set of all its eigenvalues.
For instance, an eigenvector of a rotation in three dimensions is a vector located within the axis around which the rotation is performed. The corresponding eigenvalue is 1 and the corresponding eigenspace contains all the vectors parallel to the axis. As this is a one-dimensional space, its geometric multiplicity is one. This is the only eigenvalue of the spectrum (of this rotation) that is a real number.
See also: EigenplaneExamples
As the Earth rotates, every arrow pointing outward from the center of the Earth also rotates, except those arrows that lie on the axis of rotation. Consider the transformation of the Earth after one hour of rotation: An arrow from the center of the Earth to the Geographic South Pole would be an eigenvector of this transformation, but an arrow from the center of the Earth to anywhere on the equator would not be an eigenvector. Since the arrow pointing at the pole is not stretched by the rotation of the Earth, its eigenvalue is 1.
Another example is provided by a thin metal sheet expanding uniformly about a fixed point in such a way that the distances from any point of the sheet to the fixed point are doubled. This expansion is a transformation with eigenvalue 2. Every vector from the fixed point to a point on the sheet is an eigenvector, and the eigenspace is the set of all these vectors.

However, three-dimensional geometric space is not the only vector space. For example, consider a stressed rope fixed at both ends, like the vibrating strings of a string instrument (Fig. 2). The distances of atoms of the vibrating rope from their positions when the rope is at rest can be seen as the components of a vector in a space with as many dimensions as there are atoms in the rope.
If one considers the transformation of the rope as time passes, its eigenvectors, or eigenfunctions, if one assumes the rope is a continuous medium, are its standing waves—the things that, mediated by the surrounding air, humans can experience as the twang of a bow string or the plink of a guitar. The standing waves correspond to particular oscillations of the rope such that the shape of the rope is scaled by a factor (the eigenvalue) as time passes. Each component of the vector associated with the rope is multiplied by this time-dependent factor. The amplitude (eigenvalues) of the standing waves decrease with time if damping is considered. One can then associate a lifetime with the eigenvector, and relate the concept of an eigenvector to the concept of resonance.
Eigenvalue equation
Mathematically, vλ is an eigenvector and λ the corresponding eigenvalue of a transformation equation:
if theis true, where
is the vector obtained when applying the transformation to vλ.Suppose linear transformation (which means that for all scalars a, b, and vectors v, w). Consider a basis in that vector space. Then, and vλ can be represented relative to that basis by a matrix T—a two-dimensional array—and respectively a column vector vλ—a one-dimensional vertical array. The eigenvalue equation in its matrix representation is written
is awhere the juxtaposition is matrix multiplication. This is equivalent to a set of n linear equations, where n is the number of basis vectors in the basis set. In this equation both the eigenvalue λ and the n components of vλ are unknowns.
However, it is sometimes unnatural or even impossible to write down the eigenvalue equation in a matrix form. This occurs for instance when the vector space is infinite dimensional, for example, in the case of the rope above. Depending on the nature of the transformation differential equations depending on the nature of the transformation and the space to which it applies. If is a differential operator, the eigenvectors are commonly called eigenfunctions of the differential operator representing . For example, differentiation itself is a linear transformation since (if M and N are differentiable functions, and a and b are constants)
and the space to which it applies, it can be advantageous to represent the eigenvalue equation as a set ofConsider differentiation with respect to time,
. Its eigenfunctions obey the eigenvalue equation:- ,
where λ is the eigenvalue associated with the function. Such a function of time is constant if
, grows proportionally to itself if is positive, and decays proportionally to itself if is negative. For example, an idealized population of rabbits breeds faster the more rabbits there are, and thus satisfies the equation with a positive lambda.The solution to the eigenvalue equation is exponential function; thus that function is an eigenfunction of the differential operator d/dt with the eigenvalue λ. If λ is negative, we call the evolution of N an exponential decay; if it is positive, an exponential growth. The value of λ can be any complex number. The spectrum of d/dt is therefore the whole complex plane. In this example the vector space in which the operator d/dt acts is the space of the differentiable functions of one variable. This space has an infinite dimension (because it is not possible to express every differentiable function as a linear combination of a finite number of basis functions). However, the eigenspace associated with any given eigenvalue λ is one dimensional. It is the set of all functions . N0 is an arbitrary constant, the initial population at t=0.
, theSpectral theorem
Further information: spectral theoremThe spectral theorem depicts the importance of the eigenvalues and eigenvectors for characterizing a linear transformation in a unique way. In its simplest version, the spectral theorem states that, under precise conditions, a linear transformation of a vector can be expressed as the linear combination of the eigenvectors with coefficients equal to the eigenvalues times the scalar product (or dot product) of the eigenvectors with the vector on which the transformation is applied. Mathematically, it can be written as:
where real symmetric matrix or complex Hermitian matrix.
and stand for the eigenvectors and eigenvalues of . The simplest case in which the theorem is valid is the case where the linear transformation is given by aIf one defines the nth power of a transformation as the result of applying it n times in succession, one can also define polynomials of transformations. A more general version of the theorem is that any polynomial P of is equal to:
The theorem can be extended to other functions of transformations like analytic functions, the most general case being Borel functions.
Eigenvalues and eigenvectors of matrices
Computing eigenvalues and eigenvectors of matrices
Suppose that we want to compute the eigenvalues of a given matrix. If the matrix is small, we can compute them symbolically using the characteristic polynomial. However, this is often impossible for larger matrices, in which case we must use a numerical method.
Symbolic computations
Further information: symbolic computation of matrix eigenvalues- Finding eigenvalues
An important tool for describing eigenvalues of square matrices is the characteristic polynomial: saying that λ is an eigenvalue of A is equivalent to stating that the system of linear equations (A – λI) v = 0 (where I is the identity matrix) has a non-zero solution v (an eigenvector), and so it is equivalent to the determinant:
The function p(λ) = det(A – λI) is a polynomial in λ since determinants are defined as sums of products. This is the characteristic polynomial of A: the eigenvalues of a matrix are the zeros of its characteristic polynomial.
All the eigenvalues of a matrix A can be computed by solving the equation fundamental theorem of algebra says that this equation has exactly n roots (zeroes), counted with multiplicity. All real polynomials of odd degree have a real number as a root, so for odd n, every real matrix has at least one real eigenvalue. In the case of a real matrix, for even and odd n, the non-real eigenvalues come in conjugate pairs.
. If A is an n×n matrix, then has degree n and A can therefore have at most n eigenvalues. Conversely, the- Finding eigenvectors
Once the eigenvalues λ are known, the eigenvectors can then be found by solving:
An example of a matrix with no real eigenvalues is the 90-degree clockwise rotation:
whose characteristic polynomial is
and so its eigenvalues are the pair of complex conjugates i, -i. The associated eigenvectors are also not real.Numerical computations
Further information: eigenvalue algorithmIn practice, eigenvalues of large matrices are not computed using the characteristic polynomial. Computing the polynomial becomes expensive in itself, and exact (symbolic) roots of a high-degree polynomial can be difficult to compute and express: the Abel–Ruffini theorem implies that the roots of high-degree (5 and above) polynomials cannot be expressed simply using th roots. Effective numerical algorithms for approximating roots of polynomials exist, but small errors in the eigenvalues can lead to large errors in the eigenvectors. Therefore, general algorithms to find eigenvectors and eigenvalues, are iterative. The easiest method is the power method: a random vector is chosen and a sequence of unit vectors is computed as
- , , , ...
This sequence will almost always converge to an eigenvector corresponding to the eigenvalue of greatest magnitude. This algorithm is easy, but not very useful by itself. However, popular methods such as the QR algorithm are based on it.
Properties
Algebraic multiplicity
The algebraic multiplicity of an eigenvalue λ of A is the order of λ as a zero of the characteristic polynomial of A; in other words, if λ is one root of the polynomial, it is the number of factors (t − λ) in the characteristic polynomial after factorization. An n×n matrix has n eigenvalues, counted according to their algebraic multiplicity, because its characteristic polynomial has degree n.
An eigenvalue of algebraic multiplicity 1 is called a "simple eigenvalue".
In an article on matrix theory, a statement like the one below might be encountered:
- "the eigenvalues of a matrix A are 4,4,3,3,3,2,2,1,"
meaning that the algebraic multiplicity of 4 is two, of 3 is three, of 2 is two and of 1 is one. This style is used because algebraic multiplicity is the key to many mathematical proofs in matrix theory.
Recall that above we defined the geometric multiplicity of an eigenvector to be the dimension of the associated eigenspace, the nullspace of λI − A. The algebraic multiplicity can also be thought of as a dimension: it is the dimension of the associated generalized eigenspace (1st sense), which is the nullspace of the matrix (λI − A) for any sufficiently large k. That is, it is the space of generalized eigenvectors (1st sense), where a generalized eigenvector is any vector which eventually becomes 0 if λI − A is applied to it enough times successively. Any eigenvector is a generalized eigenvector, and so each eigenspace is contained in the associated generalized eigenspace. This provides an easy proof that the geometric multiplicity is always less than or equal to the algebraic multiplicity. The first sense should not to be confused with generalized eigenvalue problem as stated below.
For example:
It has only one eigenvalue, namely λ = 1. The characteristic polynomial is spanned by the unit vector , so the geometric multiplicity is only 1.
, so this eigenvalue has algebraic multiplicity 2. However, the associated eigenspace is the axis usually called the x axis,Decomposition theorem
The decomposition theorem is a version of the spectral theorem in the particular case of matrices. This theorem is usually introduced in terms of coordinate transformation. If U is an invertible matrix, it can be seen as a transformation from one coordinate system to another. In this new system the coordinates of the vector are labeled . The latter are obtained from the coordinates v in the original coordinate system by the relation and, the other way around, we have . Applying successively , and , to the relation defining the matrix multiplication provides with , the representation of A in the new basis. The columns of U being the components of the new basis vectors within the old basis set. The decomposition theorem states that, if one chooses as columns of n linearly independent eigenvectors of A, the new matrix is diagonal and its diagonal elements are the eigenvalues of A. If this is possible the matrix A is diagonalizable. An example of non-diagonalizable matrix is given by the matrix A above.
There are several generalizations of this decomposition which can cope with the non-diagonalizable case, suited for different purposes:
- the singular value decomposition, where is diagonal but U is not necessarily equal to V;
- the Jordan normal form, where where is not diagonal but of a simple form with non vanishing entries only along the diagonal and one element above;
- any matrix A can be written uniquely as A = S + N where S is diagonalizable, N is nilpotent (i.e., such that N=0 for some q), and S commutes with N (SN=NS).
- any invertible matrix A can be written uniquely as A = SJ where S is diagonalizable and J is unipotent (i.e., such that the characteristic polynomial is a power of (λ-1), and S commutes with J).
Other theorems
The spectrum is invariant under similarity transformations: the matrices A and PAP have the same eigenvalues for any matrix A and any invertible matrix P. The spectrum is also invariant under transposition: the matrices A and A have the same eigenvalues.
A matrix is invertible if and only if zero is not an eigenvalue of the matrix.
A matrix is diagonalizable if and only if the algebraic and geometric multiplicities coincide for all its eigenvalues. In particular, an n×n matrix which has n different eigenvalues is always diagonalizable.
The vector space on which the matrix acts is always the direct sum of the generalized eigenspaces (i.e., is spanned by them and they are independent). This is true of the ordinary (non-generalized) eigenspaces if and only if they are equal to the generalized eigenspaces, i.e., if and only if the matrix is diagonalizable.
The location of the spectrum is often restricted if the matrix has a special form:
- All eigenvalues of a Hermitian matrix (A = A) are real. Furthermore, all eigenvalues of a positive-definite matrix (vAv > 0 for all vectors v) are positive.
- All eigenvalues of a skew-Hermitian matrix (A = −A) are purely imaginary.
- All eigenvalues of a unitary matrix (A = A) have absolute value one.
- The eigenvalues of a triangular matrix are the entries on the main diagonal. This holds a fortiori for diagonal matrices.
Generally, the trace, or the sum of the elements on the main diagonal of a matrix, equals the sum of the eigenvalues, and the determinant equals the product of the eigenvalues (counted according to algebraic multiplicity).
Suppose that A is an m×n matrix, with m ≤ n, and that B is an n×m matrix. Then BA has the same eigenvalues as AB plus n − m eigenvalues equal to zero.
Conjugate eigenvector
A conjugate eigenvector or coneigenvector is a vector sent after transformation to a scalar multiple of its conjugate, where the scalar is called the conjugate eigenvalue or coneigenvalue of the linear transformation. The coneigenvectors and coneigenvalues represent essentially the same information and meaning as the regular eigenvectors and eigenvalues, but arise when an alternative coordinate system is used. The corresponding equation is
For example, in coherent electromagnetic scattering theory, the linear transformation A represents the action performed by the scattering object, and the eigenvectors represent polarization states of the electromagnetic wave. In optics, the coordinate system is defined from the wave's viewpoint, known as the Forward Scattering Alignment (FSA), and gives rise to a regular eigenvalue equation, whereas in radar, the coordinate system is defined from the radar's viewpoint, known as the Back Scattering Alignment (BSA), and gives rise to a coneigenvalue equation.
Generalized eigenvalue problem
A generalized eigenvalue problem (2nd sense) is of the form
where A and B are matrices. The generalized eigenvalues (2nd sense) λ can be obtained by solving the equation
The set of matrices of the form
, where is a complex number, is called a pencil. If B is invertible, then the original problem can be written in the formwhich is a standard eigenvalue problem. However, in most situations it is preferable not to perform the inversion, and solve the generalized eigenvalue problem as stated originally.
If A and B are symmetric matrices with real entries, then the eigenvalues are real. This would have not been easy to see from the second equivalent formulation, because the matrix
is not necessarily symmetric if A and B are.An example is provided by the molecular orbital application below.
Entries from a ring
In the case of a square matrix A with entries in a ring, λ is called a right eigenvalue if there exists a column vector x such that Ax=λx, or a left eigenvalue if there exists a nonzero row vector y such that yA=yλ.
If the ring is commutative, the left eigenvalues are equal to the right eigenvalues and are just called eigenvalues. If not, for instance if the ring is the set of quaternions, they may be different.
Infinite-dimensional spaces
If the vector space is infinite dimensional, it may be advantageous to define the concept of spectral values. The spectral values are the set of scalars λ for which the Green's operator, , associated to the transformation is not defined, that is such that is not invertible (i.e., the inverse transformation to does not exist).
If λ is an eigenvalue of Hilbert or Banach spaces which have no eigenvectors at all. This can be seen on the following example. The bilateral shift on the Hilbert space (the space of all sequences of scalars such that converge) has no eigenvalue but has spectral values.
, λ is also a spectral value of it. However, the reverse relation is not true: any spectral value is not an eigenvalue. There are operators onIn functional analysis, the spectrum of an operator is defined as the set of all its spectral values. This is a key concept in scattering theory.
In infinite-dimensional spaces, the spectrum of an operator can be discrete or continuous. The former case occurs if the spectrum is a countable set of scalars; the latter if not. The exponential growth or decay provides an example of a continuous spectrum and the vibrating string an example above. The hydrogen atom is an example where both type of spectra appear. The bound states of the hydrogen atom correspond to the discrete part of the spectrum while the ionization processes are described by the continuous part. Fig. 3 exemplifies this concept in the case of the Chlorine atom.
Applications
- Schrödinger equation

An example of an eigenvalue equation where the transformation Schrödinger equation in quantum mechanics
is represented in terms of a differential operator is the time-independentwhere H, the Hamiltonian, is a second-order differential operator and , the wavefunction, is one of its eigenfunctions corresponding to the eigenvalue E, interpreted as its energy.
However, in the case we only look for the bound state solutions of the Schrödinger equation, as is usually the case in quantum chemistry, we look for within the space of square integrable functions. Since this space is a Hilbert space, with a well-defined scalar product, we can introduce a basis set in which and H can be represented as a one-dimensional array and a matrix respectively. This allows us to represent the Schrödinger equation in a matrix form. (Fig. 4 presents the lowest eigenfunctions of the Hydrogen atom Hamiltonian.)
The Dirac notation often used in this context stresses the difference between the vector or state and its representation, the function . In this context one writes the Schrödinger equation
and call Observable) instead of particular representation of it in terms of differential operators. In the equation above is understood as the vector obtained by application of the transformation H to .
an eigenstate of H (sometimes written in introductory textbooks) which is seen as a transformation (see- Molecular orbitals
In quantum mechanics, and in particular in atomic and molecular physics, within the Hartree-Fock theory, the atomic and molecular orbitals can be defined by the eigenvectors of the Fock operator. The corresponding eigenvalues are interpreted as ionization potentials via Koopmans' theorem. In this case, the term eigenvector is used in a somewhat more general meaning, since the Fock operator is explicitly dependent of the orbitals and their eigenvalues. If one wants to underline this aspect one speaks of implicit eigenvalue equation. Such equations are usually solved by an iteration procedure, called in this case self-consistent field method. In quantum chemistry, one often represents the Hartree-Fock equation in a non-orthogonal basis set. This particular representation is a generalized eigenvalue problem called Roothaan equations.
- Factor analysis
In factor analysis, the eigenvectors of a covariance matrix correspond to factors, and eigenvalues to factor loadings. Factor analysis is a statistical technique used in the social sciences and in marketing, product management, operations research, and other applied sciences that deal with large quantities of data. The objective is to explain most of the variability among a number of observable random variables in terms of a smaller number of unobservable random variables called factors. The observable random variables are modeled as linear combinations of the factors, plus "error" terms.

- Eigenfaces
In image processing, processed images of faces can be seen as vectors whose components are the brightnesses of each pixel. The dimension of this vector space is the number of pixels. The eigenvectors of the covariance matrix associated to a large set of normalized pictures of faces are called eigenfaces. They are very useful for expressing any face image as a linear combination of some of them. Eigenfaces provide a means of applying data compression to faces for identification purposes.
- Tensor of inertia
In mechanics, the eigenvectors of the inertia tensor define the principal axes of a rigid body. The tensor of inertia is a key quantity required in order to determine the rotation of a rigid body around its center of mass.
- Stress tensor
In solid mechanics, the stress tensor is symmetric and so can be decomposed into a diagonal tensor with the eigenvalues on the diagonal and eigenvectors as a basis. Because it is diagonal, in this orientation, the stress tensor has no shear components; the components it does have are the principal components.
- Eigenvalues of a graph
In spectral graph theory, an eigenvalue of a graph is defined as an eigenvalue of the graph's adjacency matrix A, or (increasingly) of the graph's Laplacian matrix , where T is a diagonal matrix holding the degree of each vertex, and in , 0 is substituted for . The principal eigenvector of a graph is used to measure the centrality of its vertices. An example is Google's PageRank algorithm. The principal eigenvector of a modified adjacency matrix of the www-graph gives the page ranks as its components.
Notes
- T. W Gorczyca, Auger Decay of the Photoexcited Inner Shell Rydberg Series in Neon, Chlorine, and Argon, Abstracts of the 18th International Conference on X-ray and Inner-Shell Processes, Chicago, August 23-27 (1999).
- In this context, only linear transformations from a vector space to itself are considered.
- Since all linear transformations leave the zero vector unchanged, it is not considered an eigenvector.
References
- Roger A. Horn and Charles R. Johnson, Matrix Analysis, Cambridge University Press (1985). ISBN 0-521-30586-1 (hardback), ISBN 0-521-38632-2 (paperback).
- John B. Fraleigh and Raymond A. Beauregard, Linear Algebra (3 edition), Addison-Wesley Publishing Company (1995). ISBN 0-201-83999-7 (international edition).
- Claude Cohen-Tannoudji, Quantum Mechanics, Wiley (1977). ISBN 0-471-16432-1. (Chapter II. The mathematical tools of quantum mechanics.)
External links
- Videos of MIT Linear Algebra Course, spring 2005 - See Lecture Eigenvalues and Eigenvectors
- MathWorld: Eigenvector
- Earliest Known Uses of Some of the Words of Mathematics: E - see eigenvector and related terms
- ARPACK is a collection of FORTRAN subroutines for solving large scale eigenvalue problems
- "Eigenvalue (of a matrix)". PlanetMath.
- Online calculator for Eigenvalues and Eigenvectors
- Online Matrix Calculator Calculates eigenvalues, eigenvectors and other decompositions of matrices online