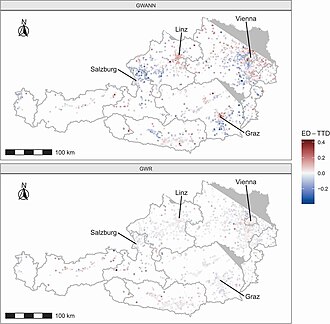
Spatial neural networks (SNNs) constitute a supercategory of tailored neural networks (NNs) for representing and predicting geographic phenomena. They generally improve both the statistical accuracy and reliability of the a-spatial/classic NNs whenever they handle geo-spatial datasets, and also of the other spatial (statistical) models (e.g. spatial regression models) whenever the geo-spatial datasets' variables depict non-linear relations.
History
Openshaw (1993) and Hewitson et al. (1994) started investigating the applications of the a-spatial/classic NNs to geographic phenomena. They observed that a-spatial/classic NNs outperform the other extensively applied a-spatial/classic statistical models (e.g. regression models, clustering algorithms, maximum likelihood classifications) in geography, especially when there exist non-linear relations between the geo-spatial datasets' variables. Thereafter, Openshaw (1998) also compared these a-spatial/classic NNs with other modern and original a-spatial statistical models at that time (i.e. fuzzy logic models, genetic algorithm models); he concluded that the a-spatial/classic NNs are statistically competitive. Thereafter scientists developed several categories of SNNs – see below.
Spatial models
Spatial statistical models (aka geographically weighted models, or merely spatial models) like the geographically weighted regressions (GWRs), SNNs, etc., are spatially tailored (a-spatial/classic) statistical models, so to learn and model the deterministic components of the spatial variability (i.e. spatial dependence/autocorrelation, spatial heterogeneity, spatial association/cross-correlation) from the geo-locations of the geo-spatial datasets’ (statistical) individuals/units.
Categories
There exist several categories of methods/approaches for designing and applying SNNs.
- One-Size-Fits-all (OSFA) spatial neural networks, use the OSFA method/approach for globally computing the spatial weights and designing a spatial structure from the originally a-spatial/classic neural networks.
- Spatial Variability Aware Neural Networks (SVANNs) use an enhanced OSFA method/approach that locally recomputes the spatial weights and redesigns the spatial structure of the originally a-spatial/classic NNs, at each geo-location of the (statistical) individuals/units' attributes' values. They generally outperform the OSFA spatial neural networks, but they do not consistently handle the spatial heterogeneity at multiple scales.
- Geographically Weighted Neural Networks (GWNNs) are similar to the SVANNs but they use the so-called Geographically Weighted Model (GWM) method/approach by Lu et al. (2023), so to locally recompute the spatial weights and redesign the spatial structure of the originally a-spatial/classic neural networks. Like the SVANNs, they do not consistently handle spatial heterogeneity at multiple scales.
Applications
There exist case-study applications of SNNs in:
- energy for predicting the electricity consumption;
- agriculture for classifying the vegetation;
- real estate for appraising the premises.
See also
- Statistics
- Neural networks' supercategories
- Statistical software
- Quantitative geography
- Spatial analysis
- GIS software
References
- ^ Hagenauer J, Helbich M (2022). "A geographically weighted artificial neural network". International Journal of Geographical Information Science. 36 (2): 215–235. Bibcode:2022IJGIS..36..215H. doi:10.1080/13658816.2021.1871618. S2CID 233883395.
- ^ Morer I, Cardillo A, Díaz-Guilera A, Prignano L, Lozano S (2020). "Comparing spatial networks: a one-size-fits-all efficiency-driven approach". Physical Review. 101 (4): 042301. Bibcode:2020PhRvE.101d2301M. doi:10.1103/PhysRevE.101.042301. hdl:2445/161417. PMID 32422764. S2CID 49564277.
- ^ Gupta J, Molnar C, Xie Y, Knight J, Shekhar S (2021). "Spatial variability aware deep neural networks (SVANN): a general approach". ACM Transactions on Intelligent Systems and Technology. 12 (6): 1–21. doi:10.1145/3466688. S2CID 244786699.
- ^ Openshaw S (1993). "Modelling spatial interaction using a neural net". In Fischer M, Nijkamp P (eds.). Geographic information systems, spatial modelling and policy evaluation. Berlin: Springer. pp. 147–164. doi:10.1007/978-3-642-77500-0_10. ISBN 978-3-642-77500-0.
- ^ Hewitson B, Crane R (1994). Neural nets: applications in geography. The GeoJournal Library. Vol. 29. Berlin: Springer. p. 196. doi:10.1007/978-94-011-1122-5. ISBN 978-94-011-1122-5.
- Openshaw S (1998). "Neural network, genetic, and fuzzy logic models of spatial interaction". Environment and Planning. 30 (10): 1857–1872. Bibcode:1998EnPlA..30.1857O. doi:10.1068/a301857. S2CID 14290821.
- Anselin L (2017). A local indicator of multivariate spatial association: extending Geary's C (PDF) (Report). Center for Spatial Data Science. p. 27.
- Fotheringham S, Sachdeva M (2021). "Modelling spatial processes in quantitative human geography". Annals of GIS. 28: 5–14. doi:10.1080/19475683.2021.1903996. S2CID 233574813.
- ^ Lu B, Hu Y, Yang D, Liu Y, Liao L, Yin Z, Xia T, Dong Z, Harris P, Brunsdon C, Comber A, Dong G (2023). "GWmodelS: A software for geographically weighted models" (PDF). SoftwareX. 21: 101291. Bibcode:2023SoftX..2101291L. doi:10.1016/j.softx.2022.101291.
- Xie Y, Chen W, He E, Jia X, Bao H, Zhou X, Ghosh E, Ravirathinam P (2023). "Harnessing heterogeneity in space with statistically guided meta-learning". Knowledge and Information Systems. 65 (6): 2699–2729. doi:10.1007/s10115-023-01847-0. PMC 9994417. PMID 37035130. S2CID 257436979.
- Rif'an M, Daryanto D, Agung A (2019). "Spatial neural network for forecasting energy consumption of Palembang area". Journal of Physics: Conference Series. 1402 (3): 033092. Bibcode:2019JPhCS1402c3092R. doi:10.1088/1742-6596/1402/3/033092. S2CID 237302678.
- Podlipnov V, Firsov N, Ivliev N, Mashkov S, Ishkin P, Skidanov R, Nikonorov A (2023). "Spectral-spatial neural network classification of hyperspectral vegetation images". IOP conference series: earth and environmental science. Vol. 1138. doi:10.1088/1755-1315/1138/1/012040.
- Lin R, Ou C, Tseng K, Bowen D, Yung K, Ip W (2021). "The Spatial neural network model with disruptive technology for property appraisal in real estate industry". Technological Forecasting and Social Change. 177: 121067. doi:10.1016/j.techfore.2021.121067.