Spectroradiometry is a technique in Earth and planetary remote sensing, which makes use of light behaviour, specifically how light energy is reflected, emitted, and scattered by substances, to explore their properties in the electromagnetic (light) spectrum and identify or differentiate between them. The interaction between light radiation and the surface of a given material determines the manner in which the radiation reflects back to a detector, i.e., a spectroradiometer. Combining the elements of spectroscopy and radiometry, spectroradiometry carries out precise measurements of electromagnetic radiation and associated parameters within different wavelength ranges. This technique forms the basis of multi- and hyperspectral imaging and reflectance spectroscopy, commonly applied across numerous geoscience disciplines, which evaluates the spectral properties exhibited by various materials found on Earth and planetary bodies.
Spectral properties such as brightness and reflectance patterns vary depending on the mineralogical compositions and crystalline structures of the given material. This variation is contributed by the presence of spectrally active components within the material, such as metallic oxides and clay minerals, which give rise to unique absorption features. Upon measurements with a spectroradiometer, these absorption features can be quantified as characteristic absorption bands in a reflectance spectra. The specific shapes associated with the bands that occur at distinctive wavelength positions enable the identification of minerals and facilitate lithological interpretations.
Conventionally, spectroradiometry is applied to the following portions of wavelengths in the electromagnetic (light) spectrum:
- Ultraviolet (UV): 1 nm – 400 nm
- Visible-near Infrared (VNIR): 400 nm – 750 nm
- Short-wave Infrared (SWIR): 750 nm – 2500 nm
- Mid Infrared (MIR): 2500 nm – 5000 nm
- Thermal Infrared (TIR): 7500 nm – 15000 nm
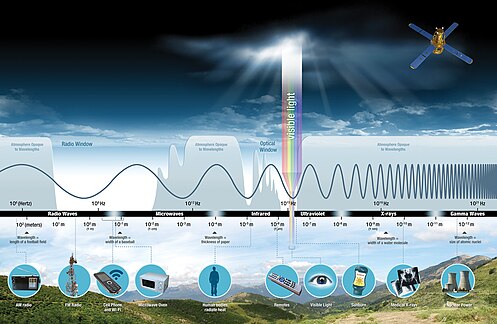
Today, most geological applications with spectroradiometry are focused within the visible-near infrared and short-wave infrared wavelength ranges. Spectroradiometry offers a simple, non-destructive, rapid, and efficient approach that complements traditional and heavy-duty geochemical methods, to characterize mineral assemblages and rock textures. It thereby facilitates the study of geological processes, exploration for natural resources, and reconstruction of past environments and climates. Its application extends not only to Earth but also to extraterrestrial planets, broadening our understanding of geological processes beyond our own planet.
Portal |
Relevant Disciplines |
Related Information |
How spectroradiometry works
In spectroradiometry, spectral features can be recognized and quantified by making use of the spectra containing different parameters measured by spectroradiometers. The most widely used spectral parameter in spectroradiometry for applications in geosciences is reflectance.
Spectroradiometry can be imaging and non-imaging in practice. Imaging spectroradiometry captures spectral data from a specific region or a scene, creating a two-dimensional image with recorded spectral information dedicated to each pixel. Non-imaging spectroradiometry, on the contrary, measures spectral data from a single point or a small focused area, offering more detailed information about the spectral properties of a specific material.
Experimental set-up
The experimental set-up for imaging spectroradiometry is simple because image processing can be conducted through computer software to show the spectral parameters for analysis, such as reflectance and brightness. Most images are freely available worldwide, widely used by different institutions, and have an extensive spatial coverage. Values of spectral parameters like reflectance can then be directly extracted from all pixels in the imagery, aggregated and averaged to produce a reflectance curve for spectral analysis.
In terms of non-imaging spectroradiometry, data collection and sampling are usually conducted through direct scanning with spectroradiometers in the laboratory or in the field. To ensure data accuracy, it is important to carry out the experiment under a stable and controlled environment. For instance, most laboratory scanning practices are performed in the dark to minimize ambient light and scattering, while field scanning is typically conducted with a contact scanning probe, such that measurements are taken in direct contact with the sample surface, free from external light sources, and in a localized setting. In both scenarios, the spectroradiometers are frequently calibrated with a white diffusion reflectance panel, which provides a reference reflectance value (99%) to maintain experimental accuracy. During spectral measurements, they exert homogeneous illumination straight towards the sample surface. Spectral data acquired will then be presented through digital software associated with the spectroradiometers.
Analysis of spectral features
Reflectance appears as individual absorption bands spanning the electromagnetic spectra, which vary with mineralogy and chemical compositions. Reflectance spectra obtained directly from the spectroradiometers without data processing are known as raw reflectance spectra. Although some prominent patterns of absorption may be identified, they are prone to influence from the overall spectra trends, and features like amplitudes and magnitudes could mislead the interpretations under such circumstances.
In order to facilitate data analysis, the raw reflectance spectra are commonly normalized to provide better visualization and quantification of trends and patterns of spectral parameters. This is commonly done by statistical techniques including detrending and continuum removal.

1. Detrending:
Removing the trend components present in the raw reflectance spectra to produce detrended spectra (usually flattened) revealing the true shapes, patterns, and distributions of absorption features.
2. Continuum removal (Convex hull transformation):
Removing overall shape, level changes, and slopes induced by other materials in the raw reflectance spectra (indicated as continuum lines) to produce continuum-removed spectra which allows effective comparison of the individual absorption features under a common baseline.
From the normalized spectra, spectral features can be accurately identified, analyzed, and compared to that among different materials. Spectrograms can also be generated using the normalized spectra to further enhance visualization. The features can be characterized using geometrical parameters describing the shapes and appearance of a particular reflectance spectrum:

- Position (P): The position of absorption band at a certain wavelength interval.
- Depth (D): The depth of absorption band (absorption value) at a certain wavelength interval.
- Width (W): The width of absorption band at a certain wavelength interval.
- Full width at half maximum (F): The width of an imaginary horizontal line positioned at half of the maximum absorption strength of a particular absorption band.
- Asymmetry (AS): The asymmetry of shape with respect to an absorption band. It can be quantified by the ratio between left and right widths with respect to the half maximum. Hence, an AS value of 1.0 will represent a symmetrical absorption band, while AS < 1.0 and AS > 1.0 will indicate leftward and rightward asymmetry.
The investigation of spectral features is often followed by building spectral indices to characterize specific minerals, i.e., parameterizing. The indices are based on the unique spectral properties exhibited by the materials, such as the positions and depths of absorption bands. A similar example of such indices is the Normalized difference vegetation index (NDVI).
Mineral identification
Minerals are identified with spectroradiometry by examining their spectral response to incoming radiation, such as brightness and reflectance, across different wavelengths. In particular, absorption bands observed in the reflectance spectra can be unique towards different minerals which allow the differentiation between one another. These absorption features arise from the distinct electronic and vibrational processes associated with the energy, molecular components, and internal structures of minerals. Electronic processes of minerals comprise charge transfers, crystal field effects (electrons achieving higher energy states), conduction bands, and colour centres, whereas vibrational processes in minerals involve stretching, bending, and rotation, which are influenced by the functional groups present in the minerals.
For example, water-bearing minerals commonly share distinctive absorption features indicating the presence of hydroxyl groups (-OH) and molecular water (H2O), which include the asymmetrical absorption features due to overtones near 1400 nm (AS1400), as well as absorption peaks near 1900 (D1900) and 2200 nm (D2200). With higher molecular water contents, the AS1400 feature becomes more asymmetrical, the absorption near 2200 nm strengthens, but the one near 1900 nm weakens. Hence, the asymmetrical absorption feature (AS1400), together with the ratio between absorption depths near 2200 and 1900 nm (D2200/D1900) are used a parameter to quantify water contents.
In practice, however, certain minerals may exhibit absorption features that coincide with those of water in similar wavelength intervals. This can potentially lead to the overlapping or masking of absorption features associated with the original minerals. Such situations may arise during field scanning or when dealing with wet samples, introducing confusion in mineral identification. Therefore, to minimize such interference, drying of samples prior to spectral scanning for mineral identification is essential. The drying process should be conducted at a temperature of 105 °C or below, which can ensure the removal of adsorbed water without causing any disruption to the internal structures of minerals.
Considering the identification capabilities of spectroradiometry for different minerals and rocks, the comprehensive databases that encompass spectral signatures are crucial. Such databases serve as valuable resources which contributes to advancing our understanding and characterization of Earth materials. Notably, the United States Geological Survey (USGS) spectral library and the ECOSTRESS spectral library represent present examples of such databases. The USGS spectral library provides a collection of reflectance spectra for rock-forming minerals and other Earth materials, spanning from ultraviolet (350 nm) to shortwave infrared (SWIR) regions (2500 nm). Likewise, the ECOSTRESS spectral library integrates spectral data from multiple spectral libraries, consolidating information on minerals and rocks into a standardized data format. These spectral libraries serve as essential references for ongoing research on spectroradiometry, providing a solid foundation for data analysis and interpretation.
Geomorphology and surface mapping
Geomorphology is the study of geological processes occurring at the surface of Earth that subsequently shape different kinds of landforms. Surface mapping is a common approach to understand these processes and their effects, which can be done by using spectroradiometry. Chemical weathering is one of the dominant processes controlling the morphology of Earth's surface, which produces iron oxides as coatings on particle surfaces, as well as clay minerals that evolve from hydrothermal alteration and decomposition of feldspars in surface soils and granitic rock bodies. These minerals are sensitive to spectral parameters like brightness and reflectance, and they exhibit distinctive absorption features on reflectance spectra, which facilitates easy diagnosis and determination of weathering states.
(1) Clay minerals (Phyllosilicates)
- Illite: Narrow absorption peaks at 1400, 1900, 2200, and 2300 nm.
- Chlorite: Triple absorption features near 2300 nm.
- Vermiculite: Pairs of narrow absorption peaks situated at 1400 and 1900 nm; weak absorption features present near 2200 and 2300 nm.
- Smectite: Strong and sharp absorption features at 1400, 1900, and 2200 nm; The peaks at 1400 and 1900 nm comes with weak absorption features attached to their right sides.
- Kaolinite: Doublet absorption peaks near 1400 and 2200 nm.
The genesis of clay minerals occurs in a progressive sequence, starting with illite and chlorite, then vermiculite, smectite, and finally forming kaolinite. Kaolinite, being the ultimate product of clay minerals, represents the most advanced stage of weathering.

(2) Iron oxides
- Hematite: Broad absorption features near 500 and 920 nm.
- Goethite: Broad absorption features near 500, 700, and 920 nm.
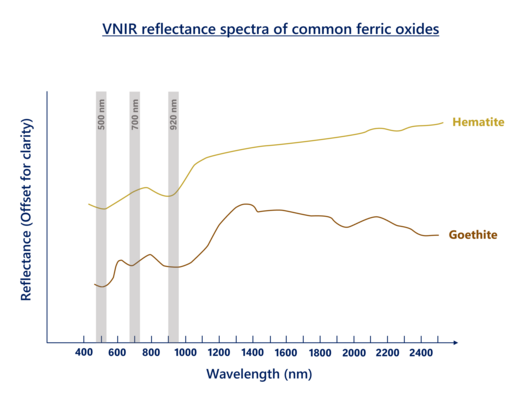
Based on the above, three groups of distinctive spectral parameters are distinguished which can serve as the indicators of weathering states.
The first group of parameters deals with the absorption features at 500, 700, and 920 nm due to ferric iron components. The peaks positioned at 920 nm (P920), and the ratio between absorption depths near 500 and 700 nm (D700/D500) are inversely proportional to the concentration of hematite, thus smaller values will mean higher degree of weathering.
The second group of parameters is related to hydroxyl group (-OH) and water (H2O). Stronger absorption absorption features near 1400 nm (AS1400), and larger ratio between absorptions near 2200 and 1900 nm (D2200/D1900), reflect higher molecular water contents, hence more alteration and weathering.
The third group of parameters concerns the effects of absorption contributed by Al-OH bonds in clay minerals including illite and kaolinite, which is situated near 2200 nm. These absorption bands become more asymmetric (AS2200 > 1, showing leftward asymmetry) with increasing kaolinite contents as a result of transformation from illite, implying greater extents of mineral alteration, degree of hydrolysis, and silicate decomposition, which serves as signals that indicate more advanced weathering stages.
Determining the above spectral parameters will enable the quantification of weathering rates, thus providing implications to paleoclimatic conditions. The application of spectroradiometry in geomorphological studies brings opportunities into rapid mapping of weathered outcrops and the study of weathering kinetics and paleoclimate particularly in remote and inaccessible regions.
Geochronology
Geochronology is the study of age and timing of geological events that have occurred throughout Earth's history. Many different geochronological methods have been developed, using various Earth materials and geological processes as proxies. Among these methods, spectroradiometry has recently emerged as a valuable tool in dating techniques, particularly in tephrochronology and surface dating applications.
Tephrochronology
Tephrochronology refers to the age determination of sedimentary strata using tephra, i.e., volcanic ashes and fragments. They serve as viable chronological markers and are precisely dated due to their instantaneous deposition over wide regions. The ashes typically have a high purity, composed of silicates (such as quartz), and phyllosilicates (such as kaolinite, serpentine), which are highly sensitive towards spectral parameters such that they demonstrate characteristic spectral features when compared to the background sediments. Volcanic ashes with a high silica content, known as felsic ashes, stand out from the background sediments due to their high albedo and reflectance values. In contrast, mafic ashes, which have low silica content, exhibit lower reflectance due to their purity compared to the mixed compositions of the background sediments. Additionally, phyllosilicate minerals in volcanic ashes display strong absorption features near 2200 nm, attributed to the stretching of hydroxyl groups (-OH bonds) with aluminium. Consequently, these spectral signatures enable the detection and differentiation of volcanic ashes from other sediments. Imaging spectroradiometry can be used for regional-scale mapping of volcanic ash deposits, as well as core logging. Meanwhile, non-imaging spectroradiometry, combined with field scanning and sampling, is suitable for localized applications, providing age implications and constraints for stratigraphic units.
Surface Dating
Surface dating is the measurement of relative age of sediment deposits on the Earth's surface. This can be achieved by utilizing weathering states as proxies, based on the principle that sediments with a higher degree of weathering have been exposed for a longer period of time. The intensity of weathering is highly correlated to the concentration of secondary iron oxides and clay minerals present in the sediments. These can be identified and measured through specific absorption features near 1400, 1900, and 2200 nm, thus establishing a relationship between age and reflectance. Using multi-, hyperspectral, and thermal imaging, the ages of surfaces of regional sediment deposits, such as alluvial fans, can be predicted and mapped.
Together, spectroradiometry provides a new approach in estimating sediment ages, as a supplement to conventional geochemical analysis. The advancement of this technique has the potential to expand surface age models to encompass remote regions, enhancing the understanding of regional geological history.
Earth resources
Earth resources are natural materials and substances that can be extracted from the Earth and used by humans for numerous purposes. Typical examples include minerals and fossil fuels. Spectroradiometry, with its ability to identify Earth materials through capturing their distinctive spectral signals, holds significant potential in exploring and predicting the presence of ore deposits and hydrocarbon reservoirs. Importantly, its applicability to inaccessible areas further expands its utility in assessing and investigating Earth's valuable resources.
Ore exploration
Spectroradiometry is widely utilized for the identification and prediction of ore deposits associated with hydrothermal systems. Hydrothermally altered deposits, known as epigenetic deposits, undergo multiple episodes of chemical alteration caused by interactions between hydrothermal fluids and the surrounding rock formations. These alteration processes are often related to volcanic and geothermal activities, where hot hydrothermal fluids penetrate through fractures in pre-existing country rocks, resulting in the deposition of valuable metallic ores such as gold and copper. Clay minerals are commonly formed as alteration products in these deposits, and their presence can be detected using spectroradiometry. Illite, for instance, is commonly observed in the vicinity of hydrothermal ore bodies. Higher concentrations of illite may indicate areas conducive to ore precipitation, and the spectral characteristics of illite, including strong absorption features near 1400, 1900, and 2200 nm (D1400, D1900, D2200) in the wavelength spectra, can be utilized to identify and trace ore fluid pathways and deposition.
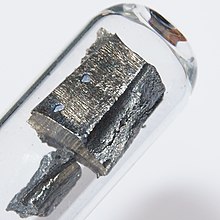
Regolith-hosted rare earth element (REE) deposits can also be identified and located using spectroradiometry. These deposits are conventionally situated in highly decomposed granitic rock bodies. The intense weathering processes occurring in granitic rocks give rise to the denudation and leaching of major element oxides, leaving behind the highly decomposed regolith. Throughout the weathering process, clay minerals such as kaolinite and halloysite are generated as alteration products, which possess a strong affinity for adsorbing REEs, leading to their enrichment in the regolith. One specific REE of interest is neodymium (Nd), which has extensive applications in the industry. Nd exhibits distinctive spectral features in reflectance spectroscopy that can be used for its detection and identification, centred near 740, 800, and 865 nm (D740, D800, D865) in the wavelength spectra. Making use of these spectral characteristics, combined with geochemical interpretations and machine learning, the identification and mapping of Nd-enriched regolith areas can be fostered, which may provide implications towards potential REE mineralization and respective ore bodies.
Hydrocarbon exploration
Most hydrocarbon reservoirs are situated deep underground, but their presence can be inferred from surface manifestations including micro-seepages. Micro-seepage occurs when hydrocarbon compounds, such as oil and gas, are released from small fractures and fissures, either directly to the ground surface, or indirectly through the impacts of volatile hydrocarbons on plants and vegetation. While micro-seepages are often not visually discernible, they can be detected using hyperspectral imaging and reflectance spectroscopy. Similarly, the same approach can be used towards the identification of coal reservoirs through their associated coal-bearing rocks, based on the unique spectral imprints given by hydrocarbons, which spans the infrared wavelength regions. Some of the signature spectral features of hydrocarbon molecules are as follows:
- OH and CH bonds: Absorption features near 1400 nm.
- Aromatic groups: Absorption features near 3280, 5250, 6200, 11000, 14000 nm.
- Aliphatic groups: Broad absorption features between 1600 – 1800 nm, 2300 – 2350 nm, and 3400 – 3500 nm, accompanied by another absorption near 6800 nm.
- Moisture contents: Absorption features near 1900 and 2940 nm.
With spectroradiometry, the spectral properties related to hydrocarbons can be easily detected and analyzed, thereby facilitating the mapping and exploration of such energy resources.
Planetary geology
The study of planetary geology looks into the geology of planets aside from Earth, as well as moons, asteroids, and other celestial bodies. Terrestrial planets have gained popularity among modern scientific research, since they offer insights into the evolution of planets and have demonstrated the potential for extraterrestrial life in the Solar System. Spectroradiometry, with its ability to characterize surficial compositions and study the geology of these celestial bodies, is considered a key technique in planetary science.
In recent years, huge efforts are devoted to the exploration of Mars, especially on its geology, which helps unravelling the planet's evolutionary history, understanding past and ongoing events occurring on the planet, and providing insights towards its habitability for human exploration.
Mars
Minerals on Mars are largely mafic, accompanied by substantial amount of clays. Each of these minerals are found in different regions on Mars, and are detected by spectroradiometers through their characteristic absorption features on the reflectance spectra.
(1) Ices
Ices composed of water and carbon dioxide are typically found in the northern and southern permanent polar caps on Mars. They also exist as seasonal frosts and clouds.
- Crystalline H2O ice: Broad absorption features near 1500 nm.
- CO2 ice: Narrow absorption features near 1435 nm, with minor absorption peaks near 2280 nm.

- Olivine: Broad absorption features centred near 1000 nm. The features get deeper and wider with increasing iron contents.
- Pyroxene: Broad absorption features near 1000 and 2000 nm. Most pyroxenes in Mars are calcium depleted (dunite, pyroxenite) which slightly shifts the absorption features towards shorter wavelengths.
- Plagioclase: Broad absorption features centred near 1300 nm given that there is substitution of iron and calcium ions.
The mafic silicates made up the composition of basaltic crusts on Mars. At Martian valleys and craters, such minerals are often seen associated with hydrated silica deposits resulted from alteration.
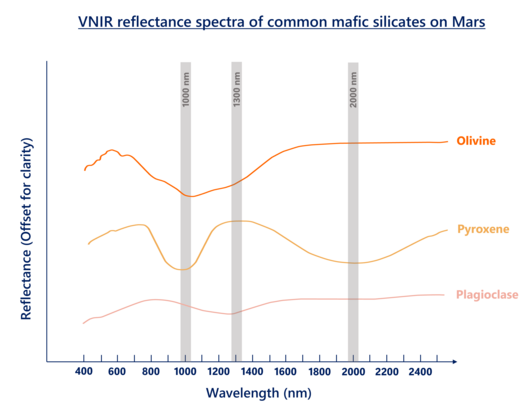
(3) Iron oxides
Iron oxides (mostly hematite) are involved in the compositions of most surface soils and dust on Mars, providing implications to Martian surficial processes.
- Hematite: Broad absorption features near 500 and 920 nm.
(4) Clay minerals (Phyllosilicates)
Iron- and magnesium-bearing clay minerals have widespread compositions on Mars. Typical examples are as follows:
- Talc: Narrow absorption features at 1400 nm, strong absorption bands near 2310 and 2390 nm.
- Prehnite: Absorption features near 1480 and 2350 nm.
- Serpentine: Broad absorption peaks centred near 1390, 2320, and 2510 nm, with a weak absorption band near 2100 nm.
Aluminium-bearing clays are also found on Mars. Their spectral characteristics are mentioned in the previous sections.


(5) Sulphates
Sulphates on Mars are polyhydrated. They are scattered along the western hemisphere, equatorial and northern part of Mars, at Valles Marineris, Meridiani Planum, and Arabia. Examples of Martian sulphates include gypsum, bassanite, kieserite, jarosite, and alunite.
- Polyhydrated sulphates: Absorption features near 1400, 1900, and 2400 nm.
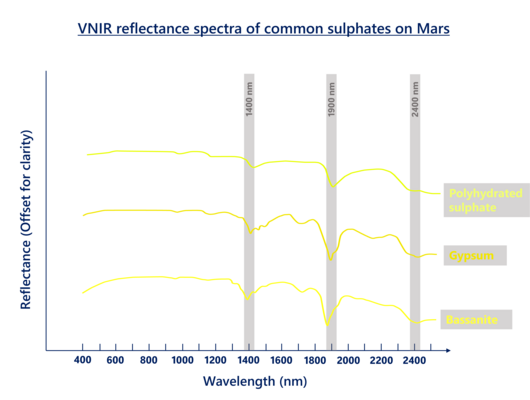
(6) Zeolites
Zeolites are identified in craters near Martian basins and highlands, providing implications towards the environments on Mars. The most distinctive zeolite mineral discovered on Mars is analcime.
- Analcime: Strong absorption features near 1790 and 2500 nm.
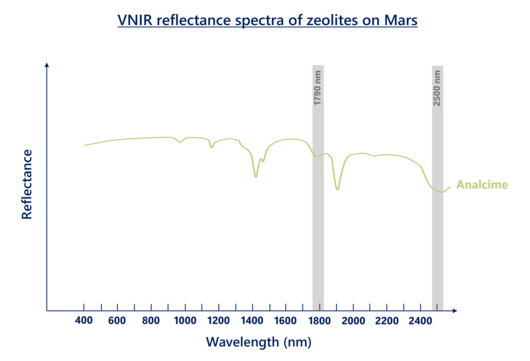
(7) Carbonates
Carbonates on Mars can be iron- or magnesium-rich. Characterized by their paired absorption features near 2300 and 2500 nm, they are found along Nili Fossae, and Tyrrhena Terra located at the southern Martian highlands.

The identification of mineral compositions on Mars offers vital clues towards Martian geological processes from the past to the present. In particular, the presence of clay minerals serves as the direct evidence of basaltic weathering on Mars. The analysis of compositional stratigraphy provided by Martian rock samples has revealed strengthening absorption features near 1400 and 1900 nm (D1400 and D1900). These features are diagnostic of elevated hydroxyl (-OH) contents owing to the increasing abundance of kaolinite, in replacement of other clay minerals. This reflects the increasing of weathering intensity and the occurrence of aqueous processes on Martian crust, indicating that a wet and warm climate had once existed on the planet.
Importantly, the occurrence of intensive chemical weathering on ancient Mars proves the past existence of water. Other than iron oxides and clays, previously detected sulphates, carbonates, and zeolites also serve as the proxies of water. Sulphates commonly form as a result of the alteration of crustal materials by groundwater and rain, and the precipitation of evaporated water bodies. Carbonates are originated from interactions between water and basalts in a CO2-rich environment, whereas zeolites are formed in alkaline waters and hydrothermal environments. The presence of these minerals altogether account for the evidence for the past occurrence of water on Mars. They also imply possibility of the planet having supported life at some point.
Spectroradiometer as a tool in Spectroradiometry
Spectroradiometers are primarily used as remote sensors in spectroradiometry to detect and quantify light intensity and its associated parameters (e.g. wavelength, amplitude). Spectral reflectance and transmittance data are digitally recorded which facilitates spectral analysis.


Components of Spectroradiometers
Main article: Spectroradiometer- Fore optics: Optical lenses, diffusers, filters, and slits. These components modify the source radiation to ensure that it can be delivered to the detectors appropriately and efficiently. A calibration unit is often attached to maintain data accuracy.
- Monochromator: Captures and splits incoming radiation (polychromatic light) into ranges of different wavelengths (monochromatic light) to foster spectral analysis.
- Detector: Detects and records spectra of electromagnetic radiation. Different detectors may be used depending on the wavelength intervals to be measured. Examples include charge-coupled device (CCD) arrays and CMOS sensors.
Imaging spectroradiometers generate digital imagery that captures spectral parameters with spatial variation, meaning that they record variations in spectral properties within the spectroradiometer's field of view. These instruments are typically larger in sizes and are situated far away from the targeted areas of measurement, such that can be found in spaceborne platforms, such as satellites, or airborne platforms, including aircraft and drones. In contrast, non-imaging spectroradiometers capture the spectral properties of the entire field of view without spatial variations. Many non-imaging spectroradiometers are relatively smaller in sizes and utilized in ground-based applications. Some are used in laboratories while some are portable and can be used in the field.
Resolution of Spectroradiometers
The resolution of spectroradiometers refers to the potential extent of details that can be detected by the sensors. In general, 4 kinds of resolutions are commonly specified for each spectroradiometer.
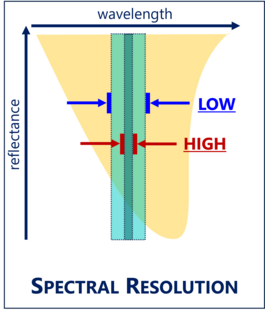
Spectral resolution concerns the capability of a sensor in a spectroradiometer to measure the light intensity according to specific wavelengths on the electromagnetic spectrum. It is related to the amount of spectral detail to be detected in each spectral band so as to discriminate among different materials. Described by the amount, wavelength interval, and width of spectral bands in which the sensor conducts wavelength measurements, a sensor with high spectral resolution would mean that it is able to capture a spectrum of light and divides it into hundreds or thousands of narrow spectral bands or channels with typical widths up to 10 and 20 nm.
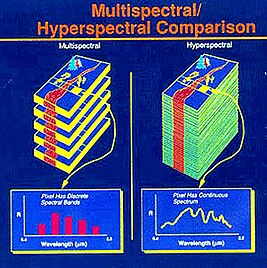
In modern times, multi- and hyperspectral imaging sensors are mainly adopted in spectroradiometry. Unlike ordinary broadband sensors which possess only a few spectral bands for measurements, they enable the extraction of spectral properties in sufficiently high spectral resolutions, allowing for the detection and analysis of diagnostic absorption features in a continuous spectrum. Hyperspectral sensors divide the detected light intensity into many, narrow, and contiguous (i.e., adjacent) spectral bands to reconstruct a full spectrum, while multispectral sensors measures light intensity using spectral bands of varying bandwidths in the wavelength spectrum which might not be contiguous. Consequently, a hyperspectral sensor is often regarded as having greater spectral resolution in comparison to a multispectral sensor, hence a better potential in mineralogical diagnosis and lithology mapping.

Spatial resolution evaluates the quality of an image captured by imaging spectroradiometers. It describes the extent of spatial detail the sensors can record, i.e., the smallest feature detected, based on pixel and grid sizes of the captured digital imagery. A sensor with fine spatial resolution would capture an image with small grid cells, thus recording more spatial details and image pixels.
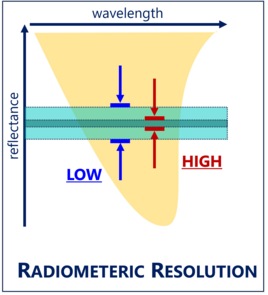
Radiometric resolution deals with the sensitivity of a sensor towards measuring the magnitude of electromagnetic radiation and light intensity. A sensor with high radiometric resolution can detect and discriminate subtle variations in brightness and radiation magnitudes. In the context of multispectral imaging, the greater the number of data bits per pixel (bit depth) of the image recorded, the better the quality and interpretability of the image, thus the finer the radiometric resolution.
Temporal resolution is the frequency or the repeat cycle of a sensor, most commonly referring to sensors on imaging spectroradiometers, to capture images and acquire spectral information. An imaging spectroradiometer with high temporal resolution typically requires less time to complete spectral measurements of an image.
Spectroradiometers in practice
The following table shows the categories and some examples of spectroradiometers worldwide which are commonly used for spectral data collection in geoscience studies.
Spectroradiometer | Category | Resolution | Primary applications |
---|---|---|---|
NASA Terra Moderate Resolution Imaging Spectroradiometer (MODIS) |
|
|
|
Advanced Spaceborne Thermal Emission and Reflectance Radiometer (ASTER) |
|
|
|
Advanced Very-High-Resolution Radiometer (AVHRR) |
|
|
|
Airborne visible/infrared imaging spectrometer (AVIRIS) |
|
|
|
Portable/ Handheld (field) spectroradiometer |
|
|
Other applications of spectroradiometry
- Ground truthing
- Soil analysis and monitoring
- Forest canopy and vegetation studies
- Landscape ecology studies
- Agricultural studies
- Biodiversity conservation
- Water quality assessments
- Camouflage characterization and detection
See also
- Spectroradiometer
- Radiometry
- Multispectral imaging
- Hyperspectral imaging
- Reflectance
- Ultraviolet-visible spectroscopy
- Infrared spectroscopy
References
- ^ van der Meer, F. D.; van der Werff, H. M. A.; van Ruitenbeek, F. J. A.; Hecker, C. A.; Bakker, W. H.; Noomen, M. F.; van der Meijde, M.; Carranza, E. J. M.; de Smeth, J. B.; Woldai, T. (2012). "Multi-and hyperspectral geologic remote sensing: A review". International Journal of Applied Earth Observation and Geoinformation. 14 (1): 112–118. doi:10.1016/j.jag.2011.08.002.
- ^ Kardeván, P. (2007). "Reflectance spectroradiometry – a new tool for environmental mapping". Carpathian Journal of Earth and Environmental Sciences. 2 (2): 29–38.
- ^ Ramakrishnan, D.; Bharti, R. (2015). "Hyperspectral remote sensing and geological applications". Current Science: 879–891.
- ^ Ghamisi, P.; Yokoya, N.; Li, J.; Liao, W.; Liu, S.; Plaza, J.; Rasti, B.; Plaza, A. (2017). "Advances in hyperspectral image and signal processing: A comprehensive overview of the state of the art" (PDF). IEEE Geoscience and Remote Sensing Magazine. 5 (4): 37–78. Bibcode:2017IGRSM...5d..37G. doi:10.1109/MGRS.2017.2762087. S2CID 24992843.
- ^ Zhao, L.; Hong, H.; Liu, J.; Fang, Q.; Yao, Y.; Tan, W.; Yin, K.; Wang, C.; Chen, M.; Algeo, T. J. (2018). "Assessing the utility of visible-to-shortwave infrared reflectance spectroscopy for analysis of soil weathering intensity and paleoclimate reconstruction". Palaeogeography, Palaeoclimatology, Palaeoecology. 512: 80–94. Bibcode:2018PPP...512...80Z. doi:10.1016/j.palaeo.2017.07.007. S2CID 133726583.
- ^ Liu, J. G.; Mason, P.J. (2016). Image processing and GIS for remote sensing: techniques and applications. John Wiley & Sons.
- ^ Tempfli, K.; Huurneman, G.; Bakker, W.; Janssen, L. L. (2009). Principles of remote sensing: an introductory textbook. International Institute for Geo-Information Science and Earth Observation.
- ^ D'Arcy, M.; Mason, P. J.; Roda-Boluda, D. C.; Whittaker, A. C.; Lewis, J. M.; Najorka, J. (2018). "Alluvial fan surface ages recorded by Landsat-8 imagery in Owens Valley, California" (PDF). Remote Sensing of Environment. 216 (401–414): 401. Bibcode:2018RSEnv.216..401D. doi:10.1016/j.rse.2018.07.013. hdl:10044/1/69550. S2CID 134468779.
- ^ Tan, W.; Qin, X.; Liu, J.; Zhou, M. F.; He, H.; Wang, C. Y.; Huang, J.; Zhu, J.; Yao, Y.; Cudahy, T. (2022). "Feasibility of visible short-wave infrared reflectance spectroscopy to characterize regolith-hosted rare earth element mineralization". Economic Geology. 117 (2): 495–508. Bibcode:2022EcGeo.117..495T. doi:10.5382/econgeo.4877. S2CID 239962247.
- ^ Leight, C. J.; McCanta, M. C.; Glotch, T. D.; Thomson, B. J.; Ye, C.; Dyar, M. D. (2022). "Characterization of tephra deposits using VNIR and MIR spectroscopy: A comprehensive terrestrial tephra spectral library". Remote Sensing of Environment. 273: 112965. Bibcode:2022RSEnv.27312965L. doi:10.1016/j.rse.2022.112965. S2CID 247289379.
- ^ Lillesand, T.; Kiefer, R. W.; Chipman, J. (2015). Remote sensing and image interpretation. John Wiley & Sons.
- ^ Haest, M.; Cudahy, T.; Laukamp, C.; Gregory, S. (2012). "Quantitative mineralogy from infrared spectroscopic data. I. Validation of mineral abundance and composition scripts at the rocklea channel iron deposit in Western Australia". Economic Geology. 107 (2): 209–228. Bibcode:2012EcGeo.107..209H. doi:10.2113/econgeo.107.2.209.
- ^ Tan, W.; Qin, X.; Liu, J.; Michalski, J.; He, H.; Yao, Y.; Yang, M.; Huang, J.; Lin, X.; Zhang, C.; Liang, X. (2021). "Visible/near infrared reflectance (VNIR) spectral features of ion-exchangeable Rare earth elements hosted by clay minerals: Potential use for exploration of regolith-hosted REE deposits". Applied Clay Science. 215: 106320. Bibcode:2021ApCS..21506320T. doi:10.1016/j.clay.2021.106320. S2CID 244065917.
- ^ Dufréchou, G.; Grandjean, G.; Bourguignon, A. (2015). "Geometrical analysis of laboratory soil spectra in the short-wave infrared domain: Clay composition and estimation of the swelling potential". Geoderma. 243: 92–107. Bibcode:2015Geode.243...92D. doi:10.1016/j.geoderma.2014.12.014.
- ^ Clark, R. N.; Swayze, G. A.; Wise, R.; Livo, K. E.; Hoefen, T. M.; Kokaly, R. F.; Sutley, S. J. (2007). "USGS digital spectral library splib06a". US Geological Survey, Data Series 231.
- ^ Aboelkhair, H.; Abdelhalim, A.; Hamimi, Z.; Al-Gabali, M. (2020). "Reliability of using ASTER data in lithologic mapping and alteration mineral detection of the basement complex of West Berenice, Southeastern Desert, Egypt". Arabian Journal of Geosciences. 13 (7): 287. Bibcode:2020ArJG...13..287A. doi:10.1007/s12517-020-5227-x. S2CID 214599580.
- ^ Goudge, T. A.; Russell, J. M.; Mustard, J. F.; Head, J. W.; Bijaksana, S. (2017). "A 40,000 yr record of clay mineralogy at Lake Towuti, Indonesia: Paleoclimate reconstruction from reflectance spectroscopy and perspectives on paleolakes on Mars". Bulletin. 129 (7–8): 806–819. Bibcode:2017GSAB..129..806G. doi:10.1130/B31569.1.
- ^ Liu, J.; He, H.; Michalski, J.; Cuadros, J.; Yao, Y.; Tan, W.; Qin, X.; Li, S.; Wei, G. J. (2021). "Reflectance spectroscopy applied to clay mineralogy and alteration intensity of a thick basaltic weathering sequence in Hainan Island, South China". Applied Clay Science. 201: 105923. Bibcode:2021ApCS..20105923L. doi:10.1016/j.clay.2020.105923. S2CID 228892175.
- ^ Fang, Q.; Churchman, G. J.; Hong, H.; Chen, Z. Q.; Liu, J.; Yu, J.; Han, W.; Wang, C.; Zhao, L.; Furnes, H. (2017). "New insights into microbial smectite illitization in the Permo-Triassic boundary K-bentonites, South China". Applied Clay Science. 140: 96–111. Bibcode:2017ApCS..140...96F. doi:10.1016/j.clay.2017.01.029.
- ^ Zhang, Y.; Tao, Q.; Komarneni, S.; Liu, J.; Zhou, Y.; Yang, F.; Zhang, B. (2021). "Clay coatings on sands in the western Qaidam Basin, Tibetan Plateau, China: Implications for the Martian clay detection". Applied Clay Science. 205: 106065. Bibcode:2021ApCS..20506065Z. doi:10.1016/j.clay.2021.106065. S2CID 233585651.
- ^ Cudahy, T.; Caccetta, M.; Thomas, M.; Hewson, R.; Abrams, M.; Kato, M.; Kashimura, O.; Ninomiya, Y.; Yamaguchi, Y.; Collings, S.; Laukamp, C.; Ong, C.; Lau, I.; Roger, A.; Chia, J.; Warren, P.; Woodcock, R.; Fraser, R.; Rankine, T.; Vote, J.; de Caritat, P.; English, P.; Meyer, D.; Doescher, C.; Fu, B.; Shi, P.; Mitchell, R. (2016). "Satellite-derived mineral mapping and monitoring of weathering, deposition and erosion". Scientific Reports. 6 (1): 23702. Bibcode:2016NatSR...623702C. doi:10.1038/srep23702. PMC 4812253. PMID 27025192.
- Niranjan, S. S.; Chaube, N.; Sarup, J. (2016). "Mapping of the carnallite mineral and sagebrush vegetation plant by using hyperspectral remote sensing and USGS spectral library". 2016 8th Workshop on Hyperspectral Image and Signal Processing: Evolution in Remote Sensing (WHISPERS): 1–5.
- ^ Hardgrove, C.; Moersch, J.; Whisner, S. (2010). "Thermal imaging of sedimentary features on alluvial fans". Planetary and Space Science. 58 (4): 482–508. Bibcode:2010P&SS...58..482H. doi:10.1016/j.pss.2009.08.012.
- ^ McCanta, M. C.; Hatfield, R. G.; Thomson, B. J.; Hook, S. J.; Fisher, E. (2015). "Identifying cryptotephra units using correlated rapid, nondestructive methods: VSWIR spectroscopy, X-ray fluorescence, and magnetic susceptibility". Geochemistry, Geophysics, Geosystems. 16 (12): 4029–4056. Bibcode:2015GGG....16.4029M. doi:10.1002/2015GC005913. S2CID 128635452.
- ^ Aymerich, I. F.; Oliva, M.; Giralt, S.; Martin-Herrero, J. (2016). "Detection of tephra layers in Antarctic sediment cores with hyperspectral imaging". PLOS ONE. 11 (1): e0146578. Bibcode:2016PLoSO..1146578A. doi:10.1371/journal.pone.0146578. PMC 4731387. PMID 26815202.
- Henderson, M. J. B.; Horgan, B. H. N.; Rowe, M. C.; Wall, K. T.; Scudder, N. A. (2021). "Determining the volcanic eruption style of tephra deposits from infrared spectroscopy". Earth and Space Science. 8 (2): e2019EA001013. Bibcode:2021E&SS....801013H. doi:10.1029/2019EA001013. hdl:2292/54968. S2CID 230607374.
- Spinetti, C.; Mazzarini, F.; Casacchia, R.; Colini, L.; Neri, M.; Behncke, B.; Salvatori, R.; Buongiorno, M. F.; Pareschi, M. T. (2009). "Spectral properties of volcanic materials from hyperspectral field and satellite data compared with LiDAR data at Mt. Etna". International Journal of Applied Earth Observation and Geoinformation. 11 (2): 142–155. Bibcode:2009IJAEO..11..142S. doi:10.1016/j.jag.2009.01.001.
- ^ Yao, Y.; Zhu, Y.; Liu, J.; Li, W. (2021). "Footprints of ore fluid pathway and implications to mineral exploration in the Shihu Gold Deposit, North China: Evidence from short wave infrared spectroscopy of illitic alteration rocks". Journal of Geochemical Exploration. 229: 106833. Bibcode:2021JCExp.22906833Y. doi:10.1016/j.gexplo.2021.106833.
- ^ Zhou, Y.; Li, L.; Yang, K.; Xing, G.; Xiao, W.; Zhang, H.; Xiu, L.; Yao, Z.; Xie, Z. (2020). "Hydrothermal alteration characteristics of the Chating Cu-Au deposit in Xuancheng City, Anhui Province, China: significance of sericite alteration for Cu-Au exploration". Ore Geology Reviews. 127: 103844. Bibcode:2020OGRv..12703844Z. doi:10.1016/j.oregeorev.2020.103844. S2CID 226346918.
- ^ Li, M. Y. H.; Zhou, M. F.; Williams-Jones, A. E. (2019). "The genesis of regolith-hosted heavy rare earth element deposits: Insights from the world-class Zudong deposit in Jiangxi Province, South China". Economic Geology. 114 (3): 541–568. Bibcode:2019EcGeo.114..541L. doi:10.5382/econgeo.4642. S2CID 164920871.
- ^ Prado, E. M. G.; de Souza Filho, C. R.; Carranza, E. J. M. (2023). "Ore-Grade Estimation from Hyperspectral Data Using Convolutional Neural Networks: A Case Study at the Olympic Dam Iron Oxide Copper-Gold Deposit, Australia". Economic Geology.
- ^ Neave, D. A.; Black, M.; Riley, T. R.; Gibson, S. A.; Ferrier, G.; Wall, F.; Broom-Fendley, S. (2016). "On the feasibility of imaging carbonatite-hosted rare earth element deposits using remote sensing". Economic Geology. 111 (3): 641–665. Bibcode:2016EcGeo.111..641N. doi:10.2113/econgeo.111.3.641. hdl:10871/19017. S2CID 39124359.
- ^ Rodrigues, S.; Fonteneau, L.; Esterle, J. (2023). "Characterisation of coal using hyperspectral core scanning systems". International Journal of Coal Geology. 269: 104220. Bibcode:2023IJCG..26904220R. doi:10.1016/j.coal.2023.104220. S2CID 257368899.
- ^ Liu, J.; Michalski, J. R.; Tan, W.; He, H.; Ye, B.; Xiao, L. (2021). "Anoxic chemical weathering under a reducing greenhouse on early Mars". Nature Astronomy. 5 (5): 503–509. Bibcode:2021NatAs...5..503L. doi:10.1038/s41550-021-01303-5. S2CID 233934224.
- ^ Qin, X.; Liu, J.; Tan, W.; He, H.; Michalski, J.; Sun, Y.; Li, S.; Ye, B.; Li, Y. L. (2023). "Characterization of kaolinized nontronite by visible to near infrared (VNIR) reflectance spectroscopy: implication for the genesis of compositional stratigraphy on Mars". Authorea Preprints.
- ^ Viviano, C. E.; Seelos, F. P.; Murchie, S. L.; Kahn, E. G.; Seelos, K. D.; Taylor, H. W.; Taylor, K.; Ehlmann, B. L.; Wiseman, S. M.; Mustard, J. F.; Morgan, M. F. (2014). "Revised CRISM spectral parameters and summary products based on the currently detected mineral diversity on Mars". Journal of Geophysical Research: Planets. 119 (6): 1403–1431. Bibcode:2014JGRE..119.1403V. doi:10.1002/2014JE004627. S2CID 16462900.
- ^ Ehlmann, B. L.; Edwards, C. S. (2014). "Mineralogy of the Martian surface". Annual Review of Earth and Planetary Sciences. 42 (1): 291–315. Bibcode:2014AREPS..42..291E. doi:10.1146/annurev-earth-060313-055024.
- ^ Fenton, L. K.; Gullikson, A. L.; Hayward, R. K.; Charles, H.; Titus, T. N. (2019). "The Mars Global Digital Dune Database (MGD3): Global patterns of mineral composition and bedform stability". Icarus. 330: 189–203. Bibcode:2019Icar..330..189F. doi:10.1016/j.icarus.2019.04.025. S2CID 164881330.
- ^ Mac Arthur, A.; MacLellan, C. J.; Malthus, T. (2012). "The fields of view and directional response functions of two field spectroradiometers". IEEE Transactions on Geoscience and Remote Sensing. 50 (10): 3892–3907. Bibcode:2012ITGRS..50.3892M. doi:10.1109/TGRS.2012.2185055. S2CID 263350939.